1
/
of
1
slot gacor
Bonanza99: Situs Slot Gacor Hari Ini Maxwin Depo 10k Terpercaya
Bonanza99: Situs Slot Gacor Hari Ini Maxwin Depo 10k Terpercaya
Regular price
Rp 10.000,00 IDR
Regular price
Sale price
Rp 10.000,00 IDR
Unit price
/
per
Couldn't load pickup availability
Bonanza99: Situs Slot Gacor Hari Ini Maxwin Depo 10k Terpercaya
Bonanza99 situs slot gacor hari ini gampang menang maxwin depo 10k di akun demo pg soft & pragmatic permainan toto dan rtp (return to player) gratis versi pro terpercaya serta alternatif bola sbobet anti rungkad terbukti triofus 2024. Memiliki raja server thailand dengan 20 link mata uang rupiah mesin mahjong ways 777 bocoran bang zeus terbukti jago deposit minimal 5000 mirip asli yang menjadi sarang buku mimpi 4d, win jackpot x500 terbaru dengan pola judi mpo atau heylink di game sangat fun seperti dewa hoki malam ini play sugar rush login mantra super joker olympus anti lag cara main 69 88 138 77 303 123 99 jp bet now.
Share
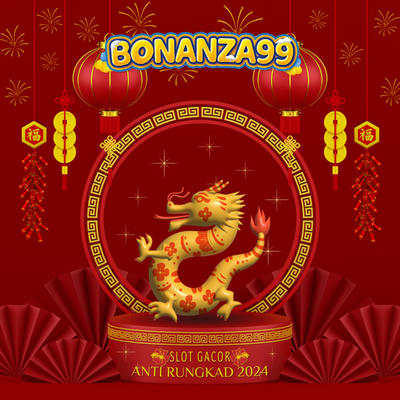